Increasing efficiency by ensuring the lowest possible consumption of materials is a big challenge for car factory owners. Read how we use machine learning to help a leading brand in the global automotive market to improve the prediction of materials consumption.
CHALLENGES
Our client, a German car manufacturer, wanted to predict daily materials consumption based on the type and number of parts sent to the production hall on a given day. The purpose of daily consumption prediction was to minimize waste and save unnecessary purchases.
The client turned to the data scientists from Stermedia to verify the idea of the internal team and refine the business side of the project. Furthermore, they expected Stermedia to conduct data analysis using artificial intelligence (machine learning).
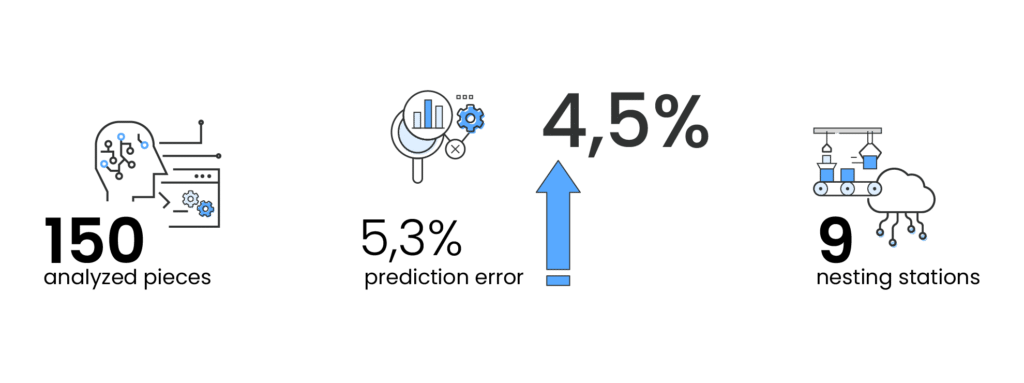
SOLUTION
Firstly, our machine learning data scientists conducted a full business interview to understand the client’s needs and choose the most adequate approach. They collected information about the inner workings of the factory, the data the customer can provide, and the process of data gathering. In the next step, they tested several models for material consumption prediction and picked the best one.
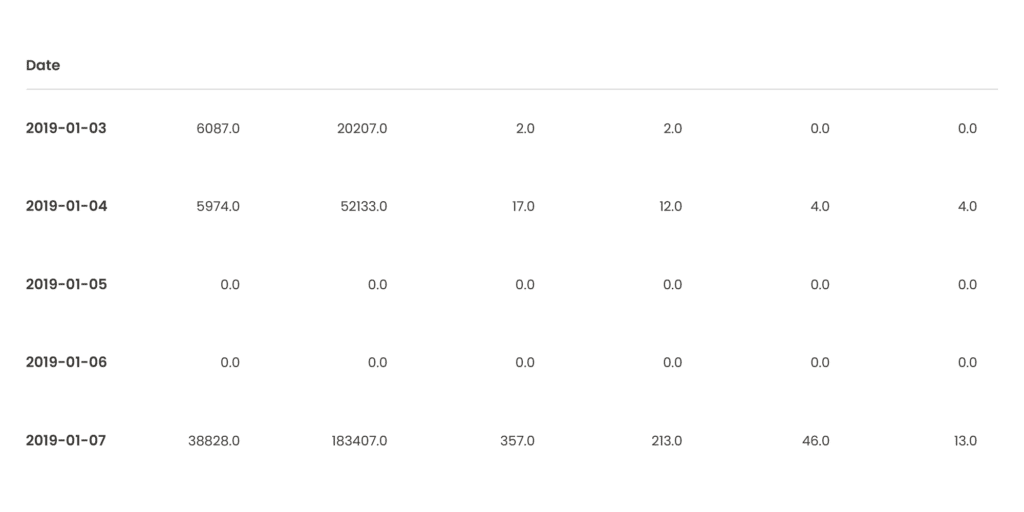
The biggest challenge our team encountered is the small amount of data the client had. The number of different types of car parts entering the factory (data columns, or features) was far greater than the number of production days (data rows, or observations). To overcome that, regularized linear models with recursive feature elimination were used. Each model was built in an iterative fashion. Starting with all available features, in every iteration step, the least important feature was being removed. The steps were as follows:
- In every iteration step, use cross-validation to check the performance of the model with the current set of features. Then, remove the feature with the lowest relevance score from the model.
- Perform the above step until there is only one feature left. Then, choose the optimal number of features by looking on the cross-validation scores obtained on all the sets of features the process went through.
The feature that enters the model was chosen with the help of correlation analysis. By the end of the process described above, each of the modeled values was assigned the optimal set of features to consider when making a prediction.
RESULTS
The plot on the left shows predicted material consumption in relation to the actual one. The closer the dots are to the diagonal, the better. In the plot on the right, the blue line is an actual material consumption, and yellow is a predicted consumption. The values are sorted for clarity. This way we can more easily focus on the days when a significant amount of material is consumed.
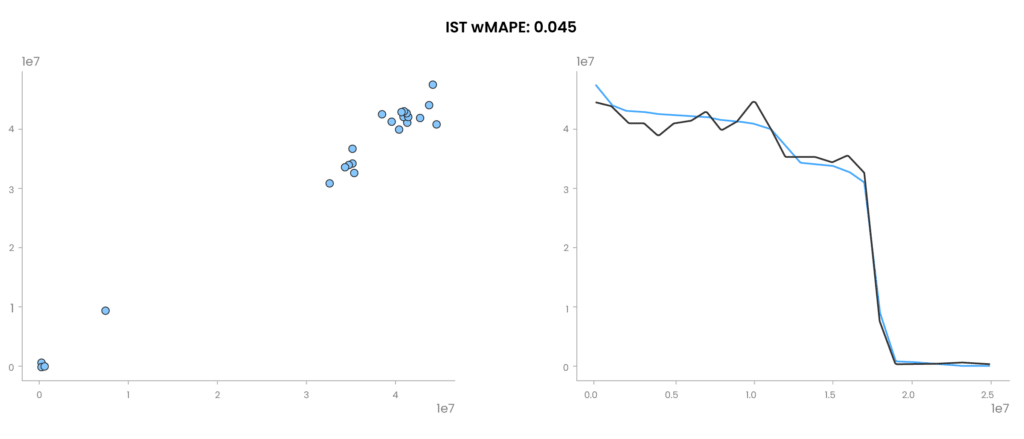
Pic. 1 Model predictions on a test dataset
It is worth mentioning that if more data were available, the team would most likely end up using random forests, neural networks, or gradient boosting methods like XGBoost or LightGBM. In this task though, classic regression models were the best choice.
As a result of this project, we improved the quality of materials consumption prediction by 15%, according to the weighted mean absolute percentage error. In a longer perspective, this prediction leads to more accurate planning and waste reduction in the manufacturing process.
TECHNOLOGIES
In the above work, the models were prepared using the Jupyter Notebook environment for Python and the scikit-learn and pandas libraries.