In recent years, Generative AI Development Services have rapidly evolved, gaining traction in areas such as AI in Software Engineering and AI and Software Development. These services are now being integrated into the software testing process, offering automation capabilities that range from generating test data to log analysis and script creation. As AI takes on a more active role in testing, an important question arises: are these advancements a supportive tool for testers, or do they signal a deeper transformation of the profession?
How Generative AI Development Services Support Software Testing
Generative AI Development Services primarily automate tasks that previously consumed significant time and manual effort. They can generate high-quality test data, write test scripts, and detect anomalies in logs faster than traditional methods. This allows testers to shift their focus toward more strategic and creative aspects of quality assurance, such as crafting edge-case scenarios or interpreting complex test results. By handling routine checks, AI significantly boosts overall testing efficiency.
Exploring the Benefits and Use Cases of Generative AI Testing
AI works particularly well in functional tests, where precision and repeatability are important. With its help, basic errors can be quickly detected and corrected efficiently. For instance, when analyzing logs, AI can spot irregularities much faster than a human. However, these results always require verification by a specialist because AI still doesn’t understand the full business context or the specifics of the application.
Can AI test non-standard scenarios?
One of the biggest challenges in software testing is so-called edge cases—unusual scenarios that may reveal hidden issues. Here, generative AI has its limitations. While it can model user behavior based on large datasets, it still lacks the intuition that allows testers to predict atypical interactions. The role of AI and Software Development in handling such scenarios is still evolving, with human expertise remaining essential.
It’s also important to note the differences between users. Younger individuals often use applications in a more dynamic way, switching between features, while older generations tend to be more linear in their approach. AI can assist in analyzing such behaviors, but without input data, it struggles to reflect the full range of possible situations.
Moreover, there is the challenge of interpreting input data by AI. For example, user behaviors may be influenced by cultural, technological, or even emotional factors. Generative AI can analyze patterns, but it requires constant data updates to adapt to changing trends
The Role of Testers in the Era of Generative AI
Generative AI is changing the approach to testing, but it does not eliminate the need for testers. Quite the opposite—they’re evolving. Instead of performing repetitive tasks, testers are becoming more analytical experts who interpret the results generated by AI. Their task also involves adapting tools to specific project requirements in AI and Software Development.
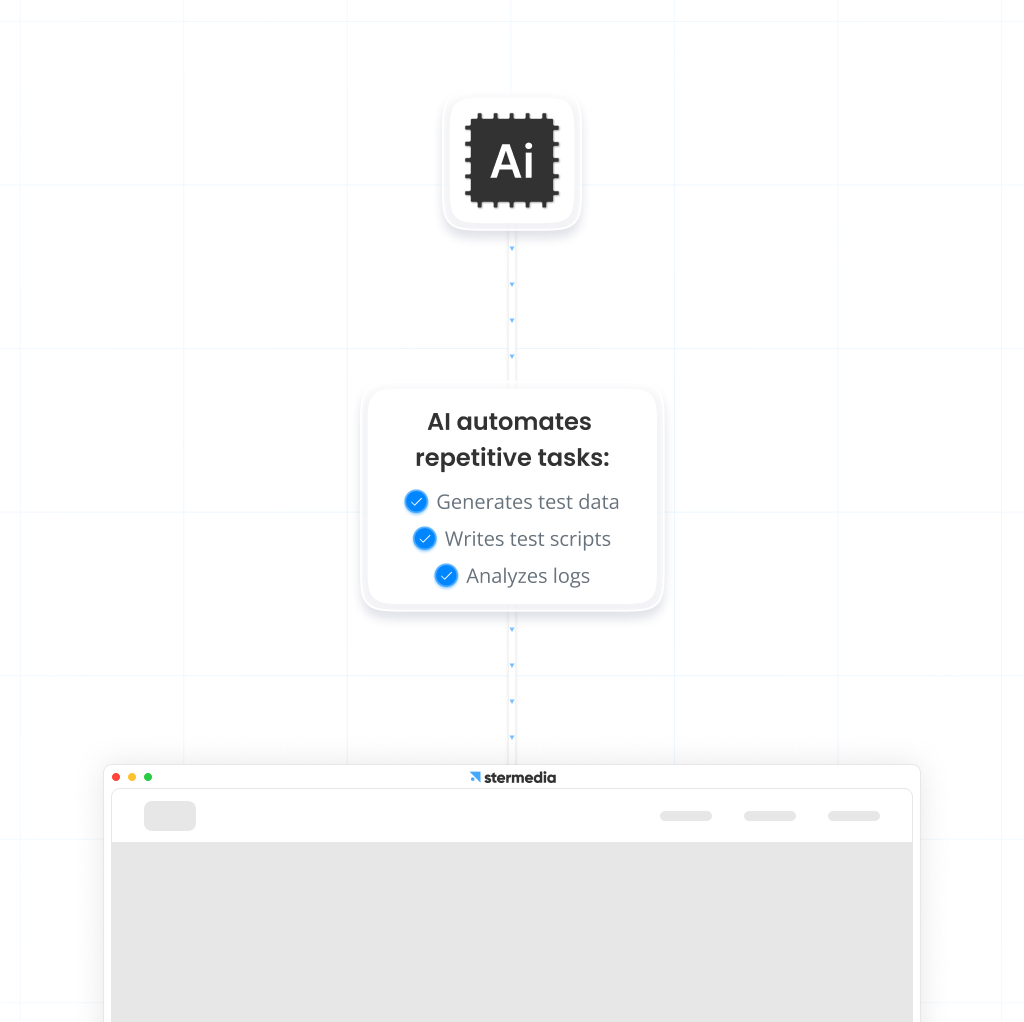
Modern testers need to use critical thinking and continuously develop their technical skills. Familiarity with AI tools, such as ChatGPT or log analysis tools, is gradually becoming standard in the industry. Therefore, education and development are crucial for those who want to keep up with changes in AI in Software Engineering.
It’s also worth mentioning that testers will increasingly be involved in the AI design process. Their knowledge of potential errors and test scenarios can be used during the training of AI models. In this way, testers can support the creation of more robust and precise tools, ensuring that AI in Software Engineering is developed with the necessary safeguards.
Risks of Generative AI Development Services in Software Testing
However, we cannot ignore the challenges. AI has a tendency to “invent” answers when it lacks sufficient data or clarity in the task. This means that results generated by AI must always be verified. Without this control, there’s a risk that errors will go unnoticed, which could have serious consequences in the real-world use of the application.
Moreover, AI can be susceptible to biases arising from the data it was trained on. This presents another task for testers—identifying and eliminating potential biases in algorithms. This is especially important in high-stakes applications, such as medicine or finance, where AI in Software Engineering must adhere to rigorous standards.
Conclusion: The Future of Testing is Collaboration
Generative AI is changing the world of testing, but it doesn’t signal the end of the tester profession. On the contrary—it opens up new opportunities and forces specialists to adapt to the new reality. Testers who can effectively collaborate with AI and harness its potential will have a competitive advantage in the job market.
The future of testing is not a competition between humans and machines, but collaboration that will lead to better, more refined software. Therefore, it’s worth viewing generative AI not as a threat, but as a tool that can significantly ease everyday work.
Generative AI, while having its limitations, offers huge potential for the IT industry’s development. Instead of fearing change, it should be seen as an opportunity for personal and professional growth. Testers who take on this challenge will find themselves at the forefront of technological advancements in AI and Software Development.
See also how generative AI supports process automation across industries in the article on Stermedia.ai: Processing car equipment using Generative AI Development.